The interaction between tumor antigens presented by major histocompatibility complex (MHC) and T-cell receptors (TCRs) is essential for activating T cells, the pivotal players in the immune response against cancer. This interaction underpins transformative cancer immunotherapies, such as immune checkpoint inhibitors and adoptive cellular therapy [1]. Understanding the molecular dynamics of these interactions is paramount for enhancing the anti-tumor immune response [2] and for surmounting resistance to TCR-based immunotherapies, thereby augmenting their efficacy in treating various cancer types [3].
In the realm of developing novel therapeutic targets and optimizing treatments, characterizing the interaction between antigens and TCRs entails two primary tasks:
Detection of immunogenic tumor antigens, especially neoantigens.
Identification of antigen-specific TCRs.
Both tasks have been challenging in contemporary research and clinical practice.
Difficulty in identification of immunogenic neoantigens and antigen-specific TCRs
The challenge is rooted in a series of intricate biological processes. Mutated proteins need to be broken down into short peptides, then transported to the endoplasmic reticulum, where they are loaded onto MHC molecules and finally brought to the cell surfaces, as depicted in Figure 1. Additionally, the interactions among antigens, MHC molecules, and TCRs exhibit polymorphic recognition patterns due to variations in the amino acid sequences of TCRs and MHC molecules among individuals. This diversity makes accurate identification even more difficult.
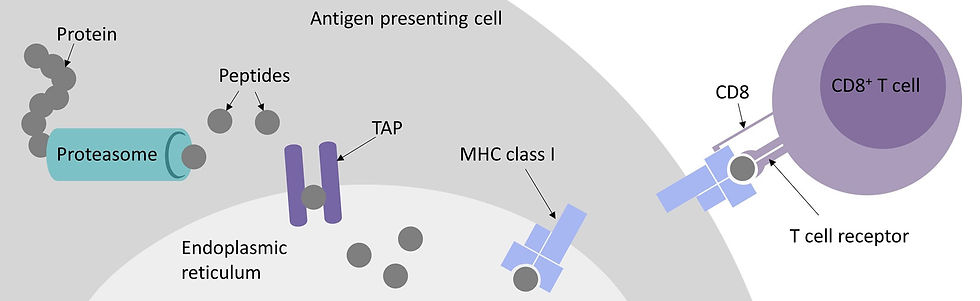
Figure 1: The antigen presentation pathway by MHC class I (MHC-I) for peptides recognized by CD8+ T cells (https://doi.org/10.3389/fgene.2019.01141)
Experimental identification typically involves sequencing expressed antigen epitopes, stimulating T cells with antigens, and then painstakingly sorting and amplifying epitope-specific T cells in vitro. However, for tumors with a high mutational burden, the experimental processes required to assess MHC binding affinity and T-cell reactivity often exceed what is feasible in real-world clinical settings [4].
The complexities involved in these processes motivate our efforts to develop AI models aimed at addressing these challenges and enhancing cancer immunotherapy by improving our ability to perform the two aforementioned tasks.
Our inaugural model, Neoantigen MUlti-taSk Tower (NeoMUST), aims to facilitate the identification of immunogenic neoantigens. Neoantigens, which emerge from mutations in tumor DNA, exhibit the potential to induce tumor-specific immune responses, thereby paving the way for the development of more efficacious and less toxic cancer treatments, including neoantigen-directed T-cell therapies and cancer vaccines. NeoMUST focuses on a key aspect of its immunogenicity: determining if neoantigens can be presented on the cell surface by MHC-I.
Contemporary approaches to predicting neoantigen presentation commonly rely on sophisticated ensemble models such as NetMHCpan4.1 and MHCflurry2.0. While these models are accurate, they demand considerable computational resources and training duration, thereby limiting accessibility for research teams with constrained resources.
To address this, we developed NeoMUST using a multi-task learning (MTL) approach. NeoMUST stands out for three reasons:
NeoMUST assimilates insights from two interconnected tasks: the prediction of antigen binding with MHC-I and its subsequent presentation on the cell surface, thereby enhancing its capability to discern both shared characteristics and distinctions between them.
NeoMUST fine-tunes its performance for each task, effectively balancing their respective loss functions.
NeoMUST achieves a notable reduction in training time, rendering it exceptionally scalable for handling large datasets.
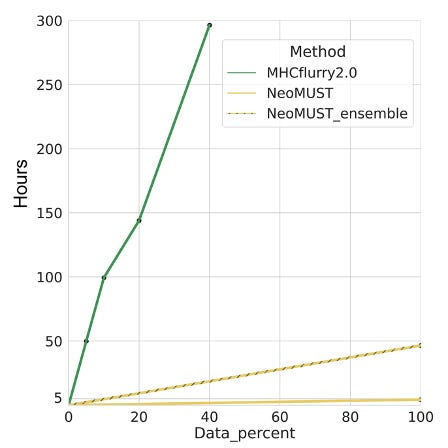
Figure 2: NeoMUST reduces the training time over 200-fold compared to MHCflurry2.0
NeoMUST delivers comparable prediction accuracy to existing models, as evidenced by its performance on comprehensive benchmark assessments. Importantly, NeoMUST achieves this level of accuracy with less than 1% of the training time needed by other models, like MHCflurry2.0, shown in Figure 2. This leap in scalability positions NeoMUST favorably for exceptional performance when trained on extensive datasets, offering heightened efficacy in scenarios involving vast data volumes. Our model with rigorous benchmark results was published in Life Science Alliance Jan 2024, 7(4).
Our latest model, TABR-BERT (TCR-Antigen Binding Recognition model based on Bidirectional Encoder Representation from Transformer), addresses the challenge of predicting interactions between TCRs, antigen peptides, and MHC molecules (TCR-pMHC), crucial for identifying antigen-specific TCRs. Accurate identification of these TCRs is pivotal for developing TCR-based therapies like TCR-engineered T cell therapy (TCR-T) [5] and TCR mimics [3], which broaden the scope of targets for cancer immunotherapy beyond surface antigens targeted by CAR-T cells.
Despite significant progress in computational identification of TCR-pMHC interactions, challenges persist due to the scarcity of labeled data and underutilization of vast unlabeled sequence data. To tackle this, TABR-BERT harnesses the Transformer architecture, akin to large language models (LLMs), to learn intricate molecular communication patterns from abundant unlabeled TCR sequences and MHC binding data. By embedding these patterns numerically, TABR-BERT predicts TCR-pMHC interactions with unparalleled accuracy, especially for unseen epitopes, illustrated in Figure 3.

Figure 3: TABR-BERT outperforms state-of-the-arts models in all benchmark tests. Panel A and B showcases the receiver operating characteristic (ROC) curves and the precision-recall (PR) curves of DLpTCR, ERGO-II, ImRex, PanPep, pMTnet, TEIM and TABR-BERT for a test set with unseen epitopes.
In an innovative application, TABR-BERT successfully identified TCRs responsive to neoantigens stemming from hotspot TP53 mutations, a pivotal tumor suppressor gene frequently mutated in various cancers [6]. This notable achievement, detailed in our publication in Briefings in Bioinformatics, Volume 25, Issue 1, January 2024, underscores TABR-BERT's potential to significantly streamline the identification of antigen-specific TCRs, thereby reducing experimental efforts and costs in cancer immunotherapy research.
In conclusion, our commitment lies in integrating AI methodologies with cancer immunotherapy. Our models are publicly accessible via our GitHub repository.Through our endeavors to analyze vast amounts of cancer genomic and clinical data, the Fresh Wind Informatics team has garnered significant expertise in several key areas:
2. Identification of antigen-reactive T-cells and TCRs
4. Single-cell sequencing data analysis to explore the tumor microenvironment (TME)
References:
1. Waldman, A.D., Fritz, J.M. & Lenardo, M.J. A guide to cancer immunotherapy: from T cell basic science to clinical practice. Nat Rev Immunol 20, 651–668 (2020). https://doi.org/10.1038/s41577-020-0306-5
2. Hosseinkhani N, Derakhshani A, Kooshkaki O, Abdoli Shadbad M, Hajiasgharzadeh K, Baghbanzadeh A, Safarpour H, Mokhtarzadeh A, Brunetti O, Yue SC, Silvestris N, Baradaran B. Immune Checkpoints and CAR-T Cells: The Pioneers in Future Cancer Therapies? Int J Mol Sci. 2020 Nov 5;21(21):8305. doi: 10.3390/ijms21218305. PMID: 33167514; PMCID: PMC7663909.
3. Chandran SS, Klebanoff CA. T cell receptor-based cancer immunotherapy: Emerging efficacy and pathways of resistance. Immunol Rev. 2019 Jul;290(1):127-147. doi: 10.1111/imr.12772. PMID: 31355495; PMCID: PMC7027847.
4. Borden ES, Buetow KH, Wilson MA, Hastings KT. Cancer Neoantigens: Challenges and Future Directions for Prediction, Prioritization, and Validation. Front Oncol. 2022 Mar 3;12:836821. doi: 10.3389/fonc.2022.836821. PMID: 35311072; PMCID: PMC8929516.
5. Tsimberidou, AM., Van Morris, K., Vo, H.H. et al. T-cell receptor-based therapy: an innovative therapeutic approach for solid tumors. J Hematol Oncol 14, 102 (2021). https://doi.org/10.1186/s13045-021-01115-0
6. Olivier M, Hollstein M, Hainaut P. TP53 mutations in human cancers: origins, consequences, and clinical use. Cold Spring Harb Perspect Biol 2010;2:a001008.
Comments